Artificial Intelligence (AI) has prevailed as transformational. Machine learning (ML) models have transformed many sectors, including public policy. Large language models (LMs) can summarize bills, extract entities and even suggest legislation.
Yet, there’s a flip side.
The risks of AI in public policy are many. AI can generate biased responses, muddy the legislative process or aid malicious actors. The intricate legislative process makes the impact of AI even harder to grasp.
The Complexity of Policymaking
Formulating a policy is a multi-year process involving many players. It takes time for grassroots activism to culminate in an executive signature. The inherently political nature of policy work further complicates reaching consensus. Bureaucracy and complexity often render the process inefficient and time-consuming. Those with power tend to reap the largest advantages.
Traditional data analysis and statistics have long influenced policy. They’re used to support data-based policies and predict bill passage and election outcomes. More recently, AI has enabled remarkable applications. ML models and natural language processing have simplified complex processes like:
- Identifying policy topics from dense legal text
- Extracting named entities such as jurisdictions, offices and agencies impacted
- Summarizing complete bill text
- Legislative research, through chat-like question answering tools
Despite its beneficial applications, the use of AI in public policy also poses risks.
Risks of AI in Public Policy
The risks of AI in public policy are many. Machine learning and statistical models can adversely affect public policy. Misunderstandings about how models work can foster distrust. Further, AI-powered tools can be intentionally misused for undemocratic goals.
AI Data Bias
Machine learning models learn from the data they’re trained on. This means that input data significantly impacts model results. As such, models train to minimize errors and maximize accuracy. Despite this, models can pick up on artifacts correlated with certain scenarios without causation.
Models that otherwise perform well may struggle with specific subgroups underrepresented in the training data, or carry negative connotations on specific groups. Researchers have discovered and categorized multiple gender, religion and ethnic biases in Reddit datasets, which are usually used when training large language models.Given the complexity, nuance and variety in biases, there’s not a single and clear solution. Google has attempted to improve face recognition tools for the Pixel 4 phone. However, this effort drew criticism surrounding data gathering methods. Addressing biases requires a multifaceted approach and strong research focus.
Privacy and Personal Information Risks
Modern ML models train on terabytes of data. It’s nearly impossible to ensure there’s no personal, private or copyrighted information in the training sets. If the dataset was not cleaned correctly, your private information may be available without your permission. Some companies might even intentionally collect this data.
There have been many cases in which law enforcement have wrongfully jailed people due to errors in face recognition models. More recently, Samsung has restricted access to ChatGPT due to leaked confidential information.AI providers are taking steps to improve data collection and use cases. OpenAI changed its policies to avoid collecting customers’ data for training by default. Many companies, including Microsoft, restrict their Face Detection models to avoid military and police uses. At the end of the day, companies will always want more data to train their models and more users to buy them. This is a constant struggle.
A Lack of Transparency in How Algorithms Work
The mathematical aspect of AI is another source of opacity. This includes linear algebra, information theory and density functions, among many complicated concepts. Neural networks, the building blocks of the best generative models, amplify this issue. With neural networks, there’s no simple interpretation of learning parameters. This results in “black box” systems, which experts find hard to trust.
Traditional openness around ML is being replaced by shallow reports and closed releases. This is due in part to the strong competition in commercial AI.
The GPT4 Technical Report is an example of the current trend in AI development. Until recently, AI providers shared state-of-the-art models and replication techniques publicly. Today, however, most state-of-the-art models are private. There’s not enough shared information to understand improvements, much less to try and replicate them. Some argue this lack of transparency prevents misuse under Responsible AI principles. Critics suggest it’s more about protecting private interests.Recent steps to increase opacity in AI have been met with pushback. This includes Meta’s commercial release of their language models. Continued research seeks to understand how models work and their impact.
Imperfect AI Models
Most state-of-the-art AI models are probabilistic. They give the most likely answer, without strict correctness, logic, or causality enforcement. It’s easy for average users to misunderstand model results and use them in the wrong situations. Dishonest actors often use this complexity for their personal gain.
Given the capabilities of LMs like GPT4 and Bard, it’s easy to believe they can solve any task. However, LMs cannot consistently solve multistep logical reasoning problems, at least for now. It’s important to understand the limitations of the models we currently have.
LMs will continue to improve. They may evolve logical and analytical capabilities in the near future. This could occur through growth in size, the improvement of world models or other techniques we don’t even know yet.
Insufficient Standards for AI Regulation
The growing complexity of ML and generative algorithms has presented roadblocks to regulation. An example of the current regulatory status is the ongoing debate around AI art. Tools like Stable Diffusion and Midjourney generate images in seconds, based on user prompts. However, the foundation of these tools is existing artists’ work. AI art tools impact the ability of artists to monetize their work, since art with similar characteristics can be created in seconds. Some argue that relying on copyright to address this issue may not be the best answer.
Around the world, governments are beginning to understand generative AI. Despite this understanding, there remains no clear path to effective regulation.
Unclear Policy Goals
The nature of public policy itself also contributes to the challenges around AI. Nuance and consensus are often hard to find in the political realm. Legislation can also be long and full of legal jargon and technicalities. Words can have many meanings — simple changes can alter the legal interpretation. Arizona SCR 1023 is a good example of how small changes can significantly affect the meaning of the law. Here’s a short paragraph changing the requirements to build a charter:
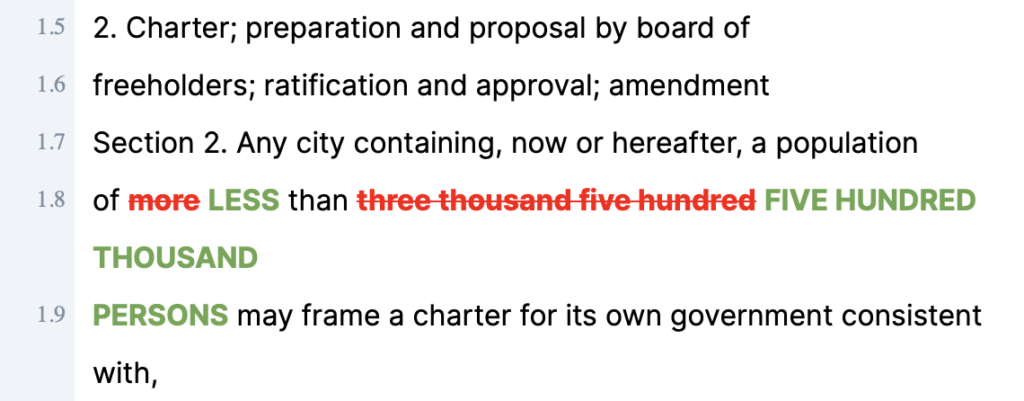
Efforts to improve AI performance in specific fields include Google’s latest release of med-palm 2. There’s even open source work around specialized legal models. Despite these efforts, more targeted resources exploring public policy and AI are needed.
AI Misuse that Undermines Democracy
Many risks of AI are born out of misunderstandings or incompetence. As such, knowledgeable actors can use AI in ways that undermine democracy. Tactics range from misleading statistics to deep-fake political images and simulated grassroots movements.
A Stanford study demonstrated how GPT3 can be used to draft persuasive political messages. Similar models have been used to generate fake reviews, or help with high school homework. A few examples of how these models could be exploited are:
- Astroturfing public interest in relevant policy topics
- Drafting bills with undemocratic objectives
- Automated email campaigns to legislators and other public officials
- Faking research or authoritative sources to support desired outcomes.
There’s a growing understanding in policy circles that AI can affect public policy. US Senators have publicly interacted with some tools. AI companies are limiting the ways their models can be used in policy settings. These efforts are not enough. AI technology exists and is being used right now. The public and private sectors need to agree on the ways AI should and should not be used to affect public policy.
Our Greatest Challenge: Mitigating Risks of AI in Public Policy
Public policy impacts us all. AI soon will, as well.
Public and grassroots pressure is needed to ensure that corporate interests align with public benefit. A great example of these efforts is Google employees’ rejection of Project Maven, Google’s contract with the Pentagon. We must also maintain incentives to continue beneficial AI research.The sluggish pace at which our government adapts can create opportunities for harmful actors and actions. Unregulated technology can impact the lives of thousands of people. Quick, effective action can often prevent harm.