One of the biggest barriers to people feeling connected to their government is a lack of understanding regarding how government works for them. Public policies (laws, regulations, rules, executive orders) are made through a complex process that involves lawmakers, advocates, lobbyists, community action and data. The policies they create and amend ends up having real impact on real people. Policy shapers use a variety of tools to make decisions about the policy they create, and with the growth of artificial intelligence technology across industries, policy shapers may be considering if they should use it as well. Here are some reasons why they should be curious about AI in improving their policymaking process.
Why Does Transparency in the Legislative Process Matter?
You may agree that laws should be created with the interest and support of the communities that are impacted. In a true democracy, every citizen’s voice is to be heard and considered in policymaking. Pluralism, in political philosophy and theory, prioritizes making room for diverse points of view in making decisions for society; it values power-sharing.
Today, rather than seeing diverse and dynamic conversation about policy, we are seeing critical issues reduced down into one of only two extreme buckets of thought and consideration. What would fix that? We need a plurality of voices to be included in the creation of policy and laws.
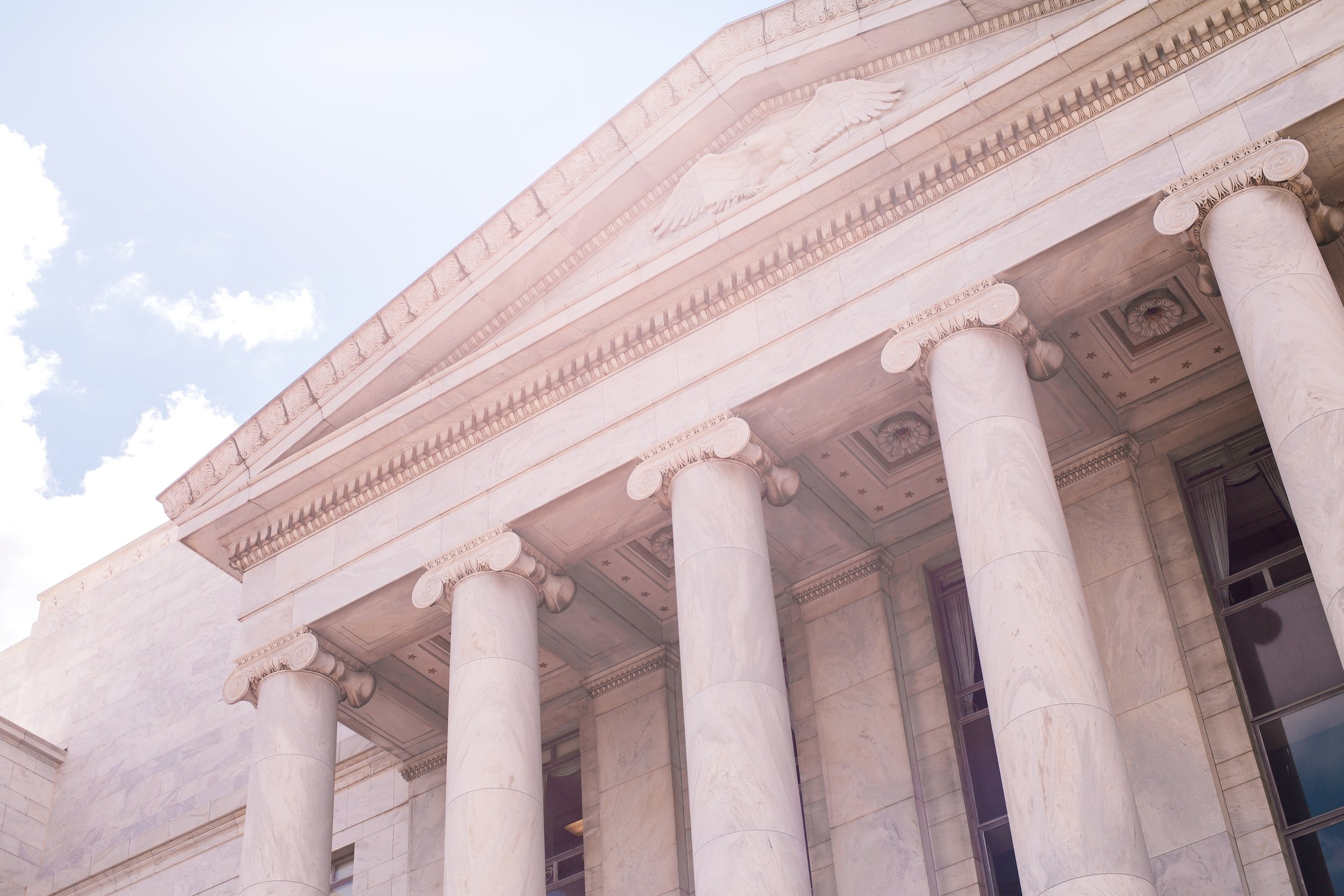
How Can AI Improve Transparency in Policymaking?
The opportunity exists for the legislative process to become more responsive and inclusive. The technology of artificial intelligence can be applied in any context; what it does is perform tasks in smart and efficient ways by learning from models that it’s given. The more data it is given to learn from, the smarter the technology gets at understanding the data and answering questions. The information that the system is learning can then be given back to us policy shapers and changemakers so that we can make better data-driven decisions in the legislative process. Here are a few examples of how AI can be applied in two policy areas: healthcare and transportation.
The Use of AI in Healthcare Policymaking
Understanding the potential impacts of various policies is a key aspect of policymaking. AI has the potential to improve healthcare-related legislation by helping policy shapers:
- Analyze health data: AI engines can analyze vast amounts of health data, including public health statistics, to identify patterns and generate insights. This can inform policy shapers on how proposed policy may contribute to health outcomes and other impacts on a community.
- Analyze healthcare costs: Assessing the cost-effectiveness of health policy and interventions can be streamlined by AI. The use of AI to calculate and model the cost of various scenarios quickly can help policymakers make better decisions about how to write policy.
- Healthcare policy research: AI can help policy shapers in communities discover which proposed legislation is being introduced and moved through legislatures across the country. Once an advocacy organization or healthcare group is aware of policy that can potentially impact their community, they can take action to help support or block that policy.
Overall, the use of AI can help policymakers make more informed decisions about healthcare policy by providing accurate, comprehensive information about the health needs of their constituents and the impacts of potential policy choices.
The Use of AI in Transportation Policymaking
As with health policy, AI has the potential to improve transportation legislation in a number of ways— here are a few examples:
- Analyze traffic patterns: AI can help policy shapers make better informed decisions by providing and quickly analyzing real-time traffic data to identify patterns and insights. Policy changes to road infrastructure, public transportation routes, and traffic laws would be written to be more effective for communities.
- Assess the environmental impact of transportation: By quickly computing complex data, AI could provide scenario modeling for different policy decisions. It could also help automakers figure out how to build their products to meet and optimize sustainability standards, for example carbon emissions.
- Transportation policy research: AI can help policy shapers and other transportation policy stakeholders, like manufacturers and drivers, make smarter decisions about which policies to support or oppose. In the US, there are laws and regulations that vary drastically state by state, and AI can help policy shapers understand that better.
Overall, AI can help lawmakers make better-informed decisions about transportation policy by providing more accurate and comprehensive information about transportation needs, potential risks and impacts, and the potential effectiveness of different policy options.
What Are the Risks Associated With the Use of AI in Public Policy?
There are always risks when trying something new, especially when technology is used in a new application. However, the government sector and its related industries are not unfamiliar with using tools and resources to create efficiencies. We can look at the application of AI in the public sector with a little less fear if we consider:
- Viewing AI as supplementary brainpower – AI allows policy experts and changemakers to think faster and with fuller data, and to have a better picture of the context in which their policy is impacting their cause. We already do this when we rely on reports from think tanks, testimonials, SMEs, etc. in developing policy. AI supercharges our ability to process data and glean valuable insights used in policymaking.
- Viewing AI as a tool, but look out for who is building the tool – AI is driven by algorithms designed by product development teams. The power of your AI tool will likely be dependent on the people and the organization that built it. Working with a vendor that you trust and that has a mission-driven approach to utilizing technology for society will likely lead to safer, more diverse and thorough outcomes when processing policy data.